时间:2024-04-26 09:30
举办地点:电气楼303
内容:
Smart Data-Driven Modeling Methods for Quality Prediction of Multigrade Processes
The development of reliable quality prediction models for multigrade industrial processes is still intractable, especially for the grades with limited quality measurements. In this work, several promising methods, including just-in-time probabilistic learning and transfer learning, have been proposed to develop smart soft sensors for the quality inferring of multigrade processes. (1) A probabilistic analysis approach using the statistical property of steady-state grades is presented for description of the current state of a new sample. Additionally, an integrated probabilistic soft sensor modeling method which can select the suitable model for quality prediction of multigrade processes has been developed. (2) By utilizing and transferring the useful information from different operating conditions to the existing soft sensor, the prediction domain is enlarged and the prediction accuracy is enhanced. Moreover, by reducing the data distribution discrepancy and enriching the information provided by the target domain, a domain adaptation-based supervised soft sensor outperforms conventional prediction models in terms of the range of prediction domains and prediction accuracy. The benefits of these soft sensors were discussed and highlighted.
主办单位:
科技处
主讲人:
刘毅,2009年6月在浙江大学获得博士学位,国家优秀青年科学基金项目获得者,浙江工业大学教授、博导。中国自动化学会和中国人工智能学会多个专委会委员,Quantitative InfraRed Thermography Journal等期刊编委。致力于过程工业数据智能领域的基础研究及应用。现主持国家基金联合重点和浙江省重大基金等项目。以第一或通讯作者在过程建模与控制领域国际知名期刊发表论文60余篇,授权10余项国家发明专利。指导2名硕士获得浙江省优秀硕士学位论文。
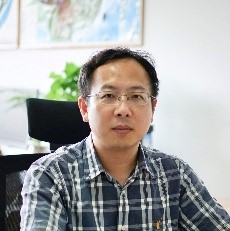